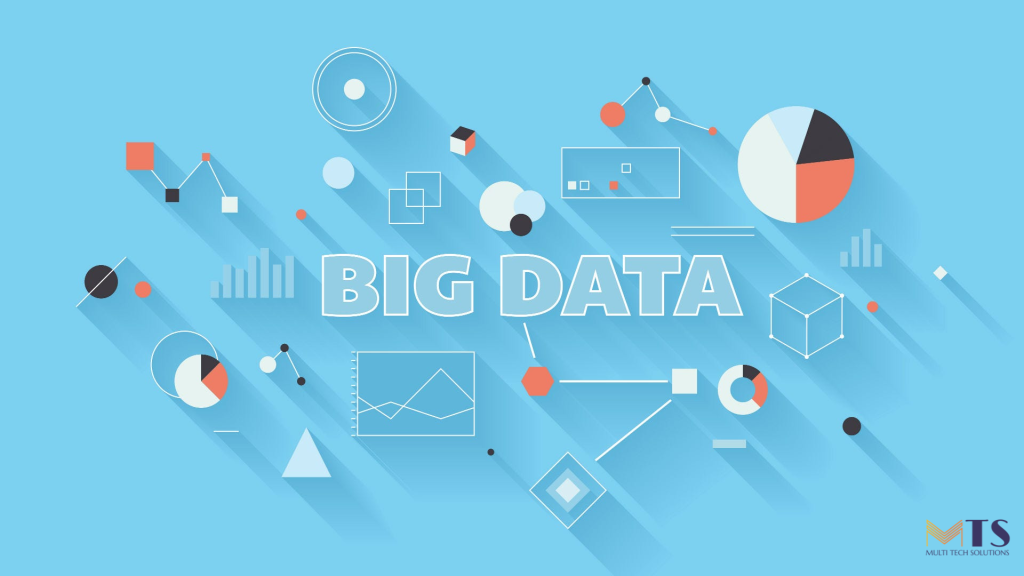
In today’s fast-paced digital age, businesses are constantly seeking ways to gain a competitive edge. One of the most valuable resources at their disposal is the vast amount of data generated daily. This treasure trove of information, commonly referred to as “big data,” holds the potential to revolutionize decision-making processes. By employing predictive analytics, organizations can unlock profound insights into future events, risks, and opportunities. In this blog post, we will delve deep into the world of predictive analytics and discover how it can be harnessed to extract meaningful business insights from big data.
Understanding Big Data
Before delving into predictive analytics, let’s get a clear understanding of what big data entails:
Definition of Big Data
Big data refers to the immense volume of structured and unstructured data that organizations accumulate from various sources. This data can be overwhelming in its size and complexity, making it challenging to process and analyze using traditional methods.
The Three V’s of Big Data
1. Volume: Big data is characterized by its sheer volume, with organizations collecting vast amounts of data daily. This includes everything from customer transactions to social media posts.
2. Velocity: Data is generated at an unprecedented speed, thanks to the proliferation of digital devices and online activities. Real-time data streams in from sources like IoT devices and social media platforms.
3. Variety: Big data comes in various forms, including structured data (like databases and spreadsheets) and unstructured data (such as text, images, and videos). This diversity adds complexity to data analysis.
4. Veracity: Data quality and accuracy are essential. Inaccurate or inconsistent data can lead to incorrect insights and decisions.
The Power of Predictive Analytics
Predictive analytics is a subset of data analytics that leverages historical data and statistical algorithms to forecast future trends, events, and outcomes. This powerful tool enables organizations to anticipate future developments and make data-driven decisions. Here’s why predictive analytics is indispensable in today’s business landscape:
1. Identifying Trends and Patterns: Predictive analytics can uncover hidden trends and patterns within big data. By examining historical data, businesses can identify recurring behaviors and make informed predictions about future occurrences. For example, a retailer can use predictive analytics to forecast consumer demand for certain products during specific seasons.
2. Mitigating Risks: Predictive analytics can help organizations assess and mitigate risks. By analyzing historical data, businesses can identify potential risks and develop strategies to mitigate them. For instance, an insurance company can use predictive analytics to assess the likelihood of policyholders filing claims, allowing them to adjust premiums accordingly.
3. Capitalizing on Opportunities: In addition to identifying risks, predictive analytics can also uncover opportunities. By analyzing customer data, organizations can identify cross-selling and upselling opportunities, leading to increased revenue. For instance, an e-commerce platform can use predictive analytics to recommend products to customers based on their purchase history and preferences.
4. Enhancing Operational Efficiency: Predictive analytics can improve operational efficiency by optimizing processes and resource allocation. For example, a logistics company can use predictive analytics to optimize delivery routes, reducing fuel consumption and delivery times.
5. Personalizing Customer Experiences: Predictive analytics plays a crucial role in delivering personalized customer experiences. By analyzing customer behavior and preferences, businesses can tailor their marketing efforts and product recommendations. This not only enhances customer satisfaction but also increases conversion rates and sales.
The Predictive Analytics Process
To harness the power of predictive analytics effectively, organizations must follow a structured process:
1. Data Collection: The first step involves collecting and aggregating relevant data from various sources. This data can include customer information, sales data, social media interactions, and more.
2. Data Preprocessing: Before analysis can begin, data must be cleaned and transformed to ensure accuracy and consistency. This process may involve removing duplicates, handling missing values, and standardizing data formats.
3. Data Analysis: In this phase, data scientists and analysts use statistical models and machine learning algorithms to analyze historical data. They look for patterns, correlations, and trends that can be used to make predictions.
4. Model Building: Once patterns are identified, predictive models are built. These models are trained on historical data and tested for accuracy and reliability.
5. Prediction and Decision-Making: With trained models in place, organizations can start making predictions about future events or outcomes. These predictions are then used to inform decision-making processes across various departments.
6. Model Deployment: Predictive models are integrated into business processes and systems to enable real-time decision-making. This can involve automating certain tasks or providing recommendations to human decision-makers.
7. Model Monitoring and Maintenance: Predictive models require regular monitoring to ensure they remain accurate and relevant. As new data becomes available, models may need to be retrained or updated to reflect changing trends.
Challenges and Considerations
While predictive analytics offers immense benefits, there are also challenges and considerations that organizations must address:
Data Privacy and Security
Dealing with big data raises concerns about data privacy and security. Organizations must ensure that sensitive data is protected and comply with relevant regulations such as GDPR.
Data Quality: The accuracy and quality of the data used for predictive analytics are paramount. Poor-quality data can lead to inaccurate predictions and unreliable insights.
Ethical Concerns: Predictive analytics can raise ethical concerns, particularly in areas like hiring and lending. Organizations must use predictive models responsibly and ensure fairness and transparency.
Model Interpretability: Understanding the rationale behind predictive model decisions can be challenging. Organizations must strive for model interpretability to build trust and make informed decisions.
In conclusion, In the era of big data, predictive analytics has emerged as a game-changer for businesses seeking to gain a competitive edge. By harnessing the power of historical data and sophisticated algorithms, organizations can unlock insights into future events, risks, and opportunities. From identifying trends and patterns to mitigating risks and personalizing customer experiences, predictive analytics has far-reaching applications. However, it’s essential to approach predictive analytics with a thoughtful and ethical mindset, ensuring data privacy, quality, and fairness.
In an increasingly data-driven world, predictive analytics is not just a luxury but a necessity for businesses aiming to thrive in the future.